Veuillez essayer un autre navigateur
Vous utilisez un navigateur obsolète qui n'est pas compatible avec le contenu de notre site Web. Pour une expérience d'affichage optimale, veuillez utiliser Microsoft Edge ou consulter notre site sur un autre navigateur.
Si vous choisissez de continuer à utiliser ce navigateur, le contenu et les fonctionnalités disponibles seront limités.

February 24, 2021
Peer into the world of business automation today and the number of different technologies is dizzying. The debate over robotic process automation (RPA) vs. artificial intelligence (AI) vs. machine learning (ML) seems to be one of the dominant conversations in this space. But is putting them in a head-to-head battle leading some businesses to miss key opportunities? To answer that question, we'll need to look at the similarities and differences in these applications.
Understanding facts such as the basic difference between RPA and machine learning reveals how each technology could best suit your business. Ultimately, we'll see that their true strength comes from a collaborative effort—and that these tools are much more interlinked than you might think.
In this blog, we'll look at the specifics of RPA vs. ML vs. AI, focusing on where these technologies differ and where they can combine to form something greater than their individual parts. Topics that we cover include:
- What are these technologies? RPA, ML and AI explained
- Leveraging the strengths of AI, ML and RPA
- Intelligent Automation: The Interlinked reality
What are these technologies? RPA, ML and AI explained
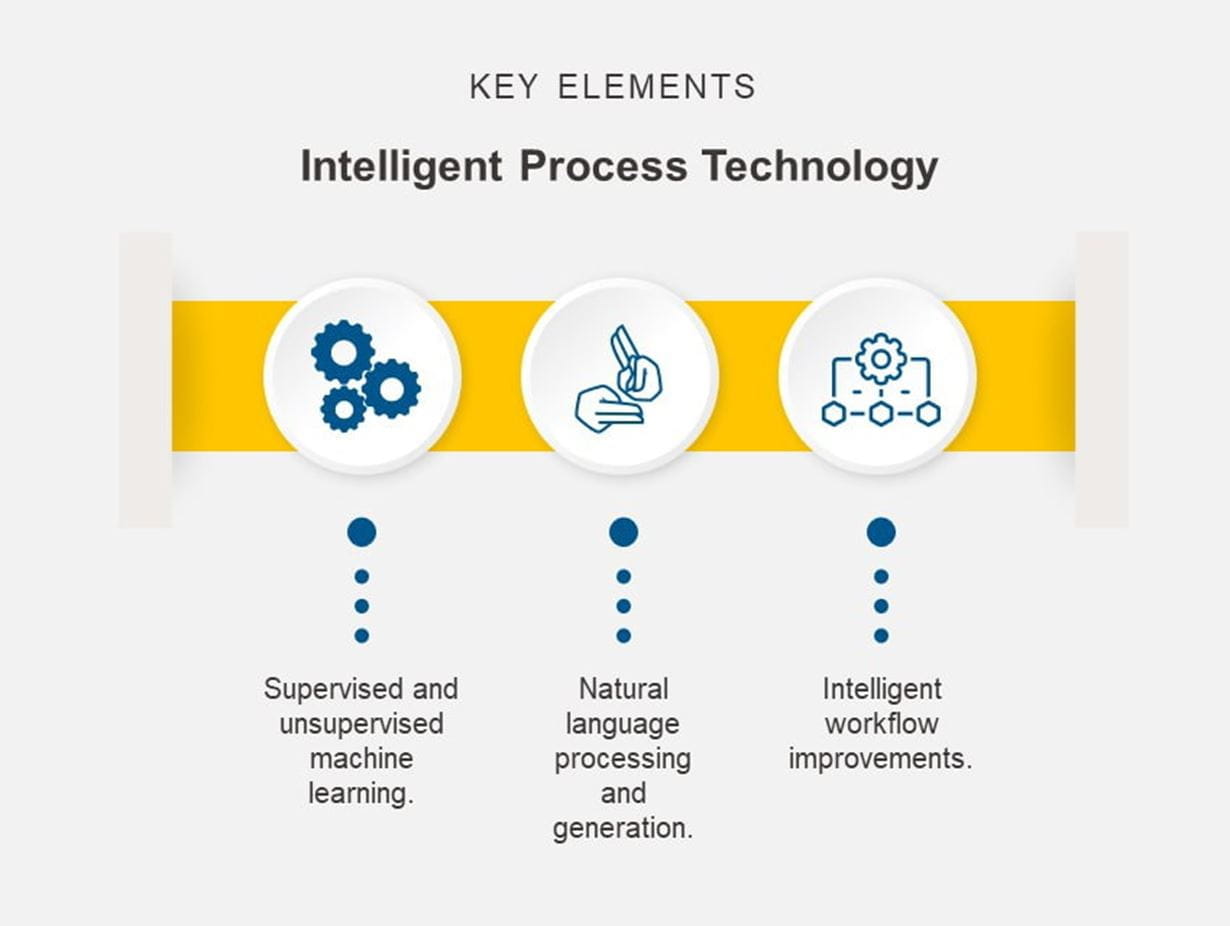
Let's begin by looking at RPA, ML and AI as separate processes. With so many initialisms and buzzwords, it's not easy to cut through the noise—but when you do, the benefits of each technology become clear.
RPA: A technology that's all about doing more with less
Robotic process automation, is where many businesses have their first encounter with advanced business technology. As a "task-oriented" automation, it has a narrow focus—it provides streamlined assistance to human workers by taking the most tedious work out of their hands.
There are a few key features of RPA to understand. RPA is:
- A strictly rule-based application. RPA software "robots" can only follow the steps and directions defined in the explicitly programmed code that makes them run.
- Excellent for task automation, such as downloading files from a web server, retrieving emails or transferring data from one program or system to another. It is a process-driven technique.
- Capable of delivering real-world business benefits. Properly configured RPA reduces cycle times, improves per-employee productivity and eliminates common error sources.
Is RPA a part of AI? It can be: These tools work together toward the same goals. However, RPA is not strictly a component of AI.
In the end, it's not a battle between RPA vs. AI because these technologies don't need to out-compete one another. Instead, they are a connected continuum of automation tools, starting from the lowest levels and progressing to advanced, process-agnostic decision-making and insight generation. They are all a part of intelligent automation.
Let's look at the next step on that staircase: machine learning vs. robotic process automation.
ML: Teaching machines to 'learn' for faster process Improvements
If RPA concerns "doing," ML concerns what its name says: learning. Typically classified as a subset of artificial intelligence, machine learning involves "training" algorithms on datasets to develop data-driven capabilities for automation. Some of the most common types of machine learning applications are analyzing large amounts of business data, recognizing patterns and using those patterns to make predictions.
Similar to RPA, there are some key features of ML to know, such as:
- ML is highly data driven, and it isn't about automating a single task within a workflow. ML and RPA can work together in these contexts, with an RPA bot taking an ML algorithm's output and transferring it to the appropriate systems.
- You can also configure ML to take actions based on things that it has learned; e.g., identifying information entered incorrectly into your systems and shifting it to the appropriate ledger. This issue can be confusing when considering RPA vs. ML, but the way that these solutions handle the process differs. RPA can only follow rules. ML applications function somewhat more independently to make decisions based on what they learn.
- One ML algorithm cannot do everything—each application has limitations based on its training data. You can't use machine learning to generate future insights from historical business sales data and then use that same algorithm to estimate tax burdens. You'll need a separately trained application for that.
Over time and with more data, ML algorithms become "smarter" as they learn how to refine their recognition of patterns. As that pattern analysis becomes more thorough and accurate, its predictive capabilities grow. ML is not only effective for identifying areas of improvement in a business process but also for transforming processes.
AI: 'Thinking' programs capable of complex workflow Automation
Finally, we've reached the biggest buzzword in business: AI. While there are no "true" AIs today—computers cannot generate novel and unique ideas on their own yet—the term is an umbrella for all "learning" technologies that simulate our own thinking patterns. This term includes not only machine learning but several other technologies, such as:
- Natural language processing, or NLP
- Deep learning and neural networks, which conduct complex analyses on unstructured data to draw conclusions
- Image and voice recognition
Within the AI umbrella, we will find techniques including both predictive and deductive analytics. As with machine learning, AI algorithms can make predictions based on the data that they ingest. However, the algorithms can also go further, deducing facts about the relationships between data. With proper oversight from its operators, AI can generate insights that offer significant opportunities to create value for the business while revolutionizing business-wide processes.
With Tungsten Automation TotalAgility®, your team can immediately begin researching multi-faceted solutions that stand at the intersection of all these tools—a position called intelligent automation. With intelligent automation through RPA with AI and ML, your business unlocks greater opportunities to realize value, improve outcomes and boost the satisfaction of your own customers. Learn more today about how Tungsten Automation RPA and TotalAgility offer today's most forward-thinking solution for automation.
Leveraging the strengths of AI, ML and RPA
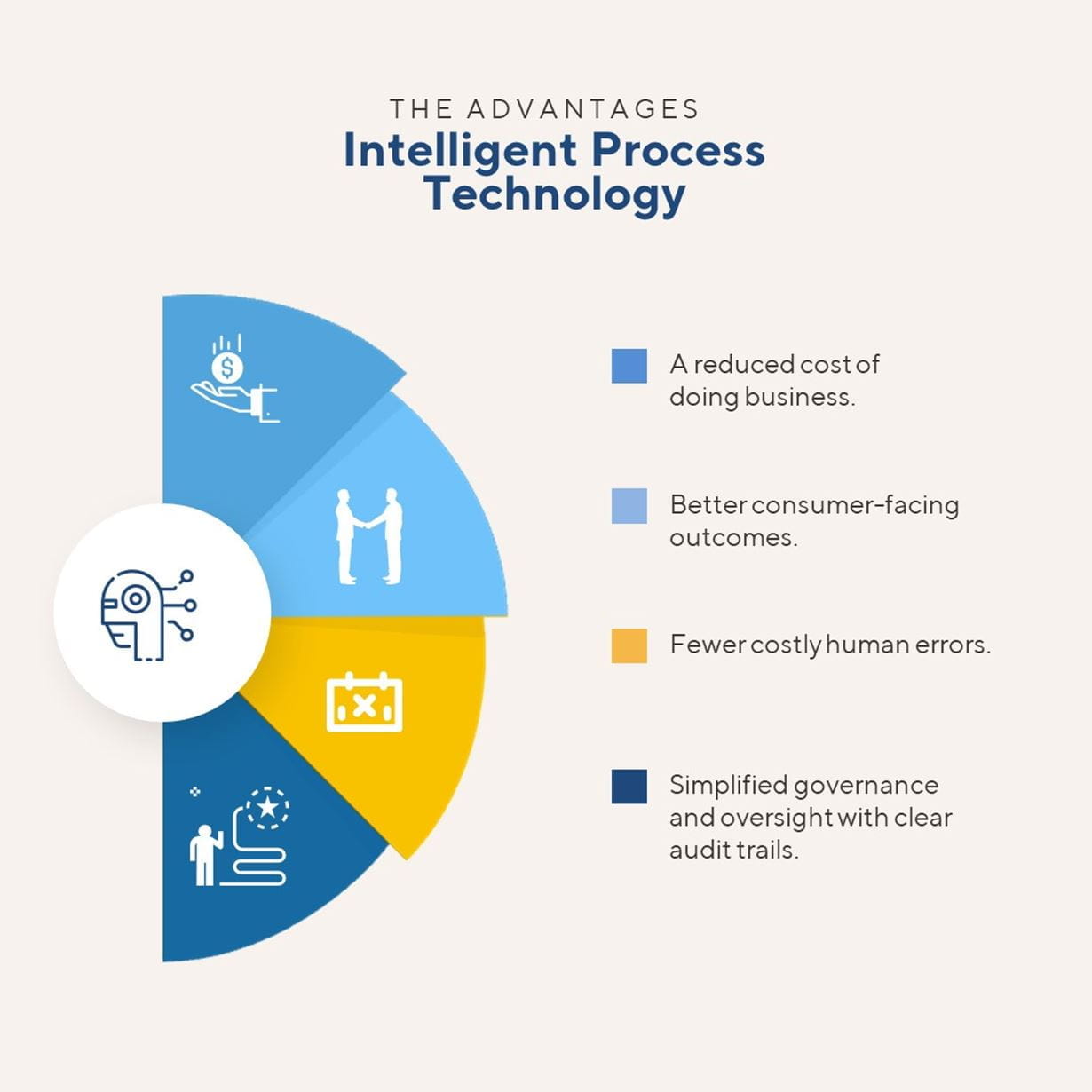
With unique strengths to each technology, how can a business use them to create better outcomes in everyday situations? By examining a few automation case studies and looking at general applications for AI, we can reveal the real-world gains that you can achieve. As we discuss these cases, notice that there is seldom only one tool at work and the use of one technology often invites the use of another.
Banks save time and money using RPA for due diligence
In finance, robotic process automation has proven itself an invaluable asset by assisting banks with regulatory compliance. Banks have a legal responsibility to conduct due diligence procedures, sometimes called "know your client," or KYC. KYC audits reveal suspicious activity that could indicate money laundering or illicit funding sources.
Banks have a requirement to take action against such accounts and to make reports—but as you might imagine, it becomes a daunting task as an institution grows and its customer base expands.
Tungsten Automation assisted a European banking partner with an intelligent automation project using RPA to slash audit times and free up a huge amount of time for employees to devote to other critical workflows. Today, after staff identify potentially problematic customer accounts, they use Tungsten Automation RPA™-configured bots to probe through the bank's systems and gather relevant data on each account. Staff then receive a report with this data ready for review, saving thousands of employee work hours each week while creating a verifiable audit trail for regulators.
This process is not only an excellent example of RPA saving a business by "doing" a task but also represents an opportunity for future growth. With an AI platform such as TotalAgility, one possibility is using ML and AI applications to make those risk assessments automatically. Bots would gather the info and feed it to the AI algorithms, which could then provide a decision for a final human review.
How a European Insurer using ML-Powered cognitive capture Improved efficiency
Insurance presents an interesting case for ML and AI because it is a work environment with a challenging amount of structured and unstructured data. One insurance business working with Tungsten Automation faced bottlenecks in claims processing due to the amount of investigating data adjusters needed to read and understand.
Using the advanced optical character recognition (OCR) technology built into the TotalAgility platform, the agency developed a system that cut their processing time by up to 80%.
Documents that staff scanned into the system went through an intelligent OCR system called cognitive capture, which uses ML to understand different document template formats. Once it recognized and identified these formats, the TotalAgility application extracted only the most relevant data from the documents and placed it within a system accessible by the customer service team.
A simple bot rapidly retrieves and displays the information to aid employees in making faster, well-informed decisions. This process is another example of the differences between RPA versus AI that also showcases how these tools work together to produce intelligent automation techniques.
RPA gathers data for AI algorithms making credit decisions
Assessing credit risks and selecting potentially profitable loan opportunities are other applications for these techniques. A business funding provider that Tungsten Automation worked with developed its own in-house predictive AI algorithms for making credit decisions. However, feeding relevant data into that tool was a challenge.
There was also a secondary challenge: the need to fully automate the process for customers. The funding provider aimed to make the process seamless from an applicant's perspective. Tungsten Automation RPA was fundamental to assisting in this effort. When a customer submits an application, hundreds of different robots go to work polling databases and extracting information from customer materials.
Upon completion, the bots feed that data back into the predictive algorithm. Using extensive training on historic data based on approved or denied applications, the AI then provides a decision—transforming a process that could take hours or days into one that finishes in minutes.
Intelligent Automation: The interlinked reality of AI, ML and RPA
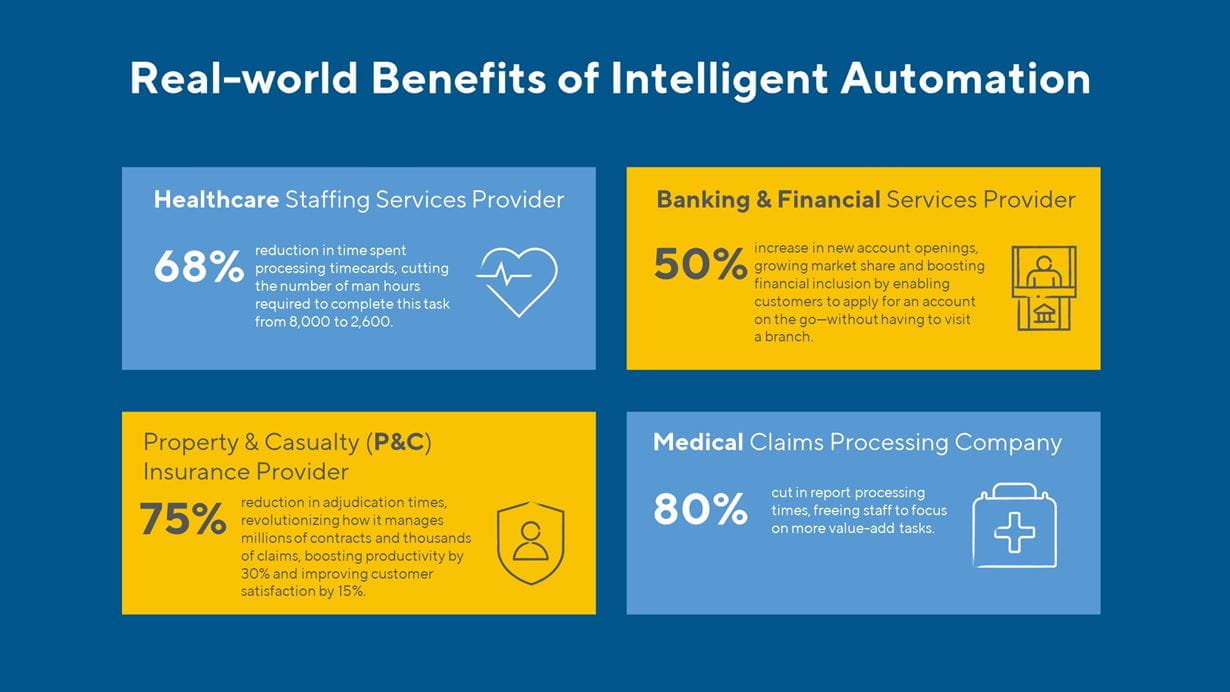
RPA, AI and ML may all refer to different technologies and automation techniques, but it's clear from these case studies that their real value doesn't lie in isolated uses. Instead, intelligent automation that shares these tools is the way forward for the businesses of tomorrow. With systems that can communicate, make decisions and translate those efforts into actionable business insights, your business gains opportunities to do more with far less.
Platforms such as TotalAgility offer a unified approach, folding multiple intelligent automation technologies into one package. With these solutions, strategizing for your company's next growth stage starts right now.
Are there opportunities in your business to make the most of the potential locked within RPA, ML and AI? Dive deeper into the world of intelligent automation today to explore the change that it could create within your company.

Leader in Intelligent Document Processing by Zinnov Zones for fifth year in a row
Télécharger le rapport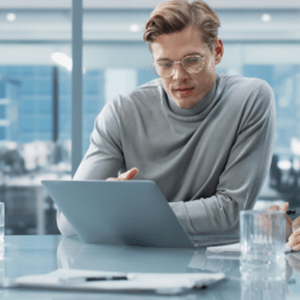
Nous contacter
Contactez un expert Tungsten Automation pour en savoir plus sur nos solutions.
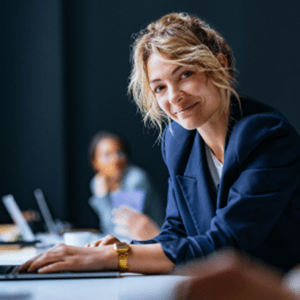
Demander une démo
Grâce à une démo personnalisée, vous découvrirez comment nous pouvons vous aider à favoriser l’innovation, augmenter votre productivité et améliorer vos résultats.